Linear regression is one of the most important methods of data analysis. It serves the determination of model parameters, model fitting, assessing the importance of influencing factors, and prediction, in all areas of human, natural and economic sciences. Computer scientists who work closely with people from these areas will definitely come across regression models.
The aim of this chapter is a first introduction into the subject. We deduce the coefficients of the regression models using the method of least squares to minimise the errors. We will only employ methods of descriptive data analysis. We do not touch upon the more advanced probabilistic approaches which are topics of statistics. For that, as well as for nonlinear regression, we refer to the specialised literature.
We start with simple (or univariate) linear regression—a model with a single input and a single output quantity—and explain the basic ideas of analysis of variance for model evaluation. Then we turn to multiple (or multivariate) linear regression with several input quantities. The chapter closes with a descriptive approach to determine the influence of the individual coefficients.
18.1 Simple Linear Regression
A first glance at the basic idea of linear regression was already given in Sect. 8.3. In extension to this, we will now allow more general models, in particular regression lines with nonzero intercept.
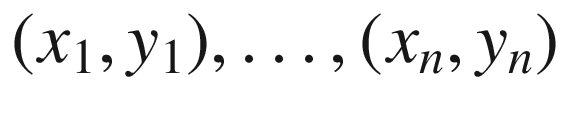












Scatter plot height/weight, line of best fit, best parabola
Example 18.1












Scatter plot height of fathers/height of the sons, regression line






Linear model and error












Proposition 18.2




Proof
With the notations and
the determinant of the normal
equations is
. For vectors of length
and
we know that
, see Appendix A.4, and thus
. This relation, however, is valid in
any dimension n (see for
instance [2, Chap. VI, Theorem 1.1]), and equality can only occur
if
is parallel to
, so all components
are equal. As this possibility was
excluded, the determinant of the normal equations is greater than
zero and the solution formula is obtained by a simple
calculation.












Linear model, prediction, residual
With the above specifications, the
deterministic regression model
is completed. In the statistical
regression model the errors are interpreted as random variables
with mean zero. Under further probabilistic assumptions the model
is made accessible to statistical tests and diagnostic procedures.
As mentioned in the introduction, we will not pursue this path here
but remain in the framework of descriptive data analysis.







Example 18.3







18.2 Rudiments of the Analysis of Variance
First indications for the quality of fit of the linear model can be obtained from the analysis of variance (ANOVA), which also forms the basis for more advanced statistical test procedures.











- (a)
The data values
themselves already lie on a straight line. Then all
and thus
,
, and the regression model describes the data record exactly.
- (b)
The data values are in no linear relation. Then the line of best fit is the horizontal line through the mean value (see Exercise 13 of Chap. 8), so
for all i and hence
,
. This means that the regression model does not offer any indication for a linear relation between the values.
The basis of these considerations is the validity of the following formula.
Proposition 18.4
(Partitioning of total
variability) .
Proof




















Remark 18.5
An essential point in the proof of
Proposition 18.4 was the property of that its first line was composed of
ones only. This is a consequence of the fact that
was a model parameter. In the
regression where a straight line through the origin is used (see
Sect. 8.3) this is not the case. For a
regression which does not have
as a parameter the variance partition
is not valid and the coefficient of determination is
meaningless.
Example 18.6



Example 18.7













|
4 |
8 |
12 |
16 |
24 |
32 |
---|---|---|---|---|---|---|
|
16 |
48 |
90 |
120 |
192 |
283 |






Fractal dimension of the coastline of Great Britain
A word of caution is in order. Data
analysis can only supply indications, but never a proof that a
model is correct. Even if we choose among a number of wrong models
the one with the largest , this model will not become correct.
A healthy amount of skepticism with respect to purely empirically
inferred relations is advisable; models should always be critically
questioned. Scientific progress arises from the interplay between
the invention of models and their experimental validation through
data.
18.3 Multiple Linear Regression








Multiple linear regression through a scatter plot in space
Example 18.8
A vending machine company wants to
analyse the delivery time, i.e., the time span y which a driver needs to refill a
machine. The most important parameters are the number of refilled product units and the
distance
walked by the driver. The results of
an observation of 25 services are given in the M-file mat18_3.m. The data
values are taken from [19]. The observations
with the corresponding service times
yield a scatter plot in space to
which a plane of the form
should be fitted (Fig. 18.6; use the M-file
mat18_4.m
for visualisation).
Remark 18.9

















Example 18.10





18.4 Model Fitting and Variable Selection





















Average
explanatory power of individual coefficients. One first
computes all possible sequential, partial coefficients of
determination which can be obtained by adding the variable
to all possible combinations of the
already included variables. Summing up these coefficients and
dividing the result by the total number of possibilities, one
obtains a measure for the contribution of the variable
to the explanatory power of the
model.
Average over orderings was proposed by [16]; further details and advanced considerations can be found, for instance, in [8, 10]. The concept does not use probabilistically motivated indicators. Instead it is based on the data and on combinatorics, thus belongs to descriptive data analysis. Such descriptive methods, in contrast to the commonly used statistical hypothesis testing, do not require additional assumptions which may be difficult to justify.
Example 18.11
















Average explanatory powers of the individual variables





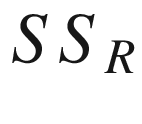








Experiment 18.12
Open the applet Linear regression and load data set
number 9. It contains experimental data quantifying the
influence of different aggregates on a mixture of concrete. The
meaning of the output variables through
and the input variables
through
is explained in the online
description of the applet. Experiment with different selections of
the variables of the model. An interesting initial model is
obtained, for example, by choosing
as independent and
as dependent variable; then remove
variables with low explanatory power and draw a pie chart.
18.5 Exercises
- 1.
-
The total consumption of electric energy in Austria 1970–2015 is given in Table 18.1 (from [26, Table 22.13]). The task is to carry out a linear regression of the form
through the data.
- (a)
-
Write down the matrix
explicitly and compute the coefficients
using the MATLAB command
.
- (b)
-
Check the goodness of fit by computing
. Plot a scatter diagram with the fitted straight line. Compute the forecast
for 2020.
Table 18.1Electric energy consumption in Austria, year
, consumption
[GWh]
1970
1980
1990
2000
2005
2010
2013
2014
2015
23.908
37.473
48.529
58.512
66.083
68.931
69.934
68.918
69.747
- 2.
-
A sample of
civil engineering students at the University of Innsbruck in the year 1998 gave the values for
height [cm] and
weight [kg], listed in the M-file mat18_ex2.m. Compute the regression line
, plot the scatter diagram and calculate the coefficient of determination
.
- 3.
-
Solve Exercise 1 using Excel.
- 4.
-
Solve Exercise 1 using the statistics package SPSS.
Hint. Enter the data in the worksheet Data View; the names of the variables and their properties can be defined in the worksheet Variable View. Go to Analyze
Regression
Linear.
- 5.
-
The stock of buildings in Austria 1869–2011 is given in the M-file mat18_ex5.m (data from [26, Table 12.01]). Compute the regression line
and the regression parabola
through the data and test which model fits better, using the coefficient of determination
.
- 6.
-
The monthly share index for four breweries from November 1999 to November 2000 is given in the M-file mat18_ex6.m (November 1999
, from the Austrian magazine profil 46/2000). Fit a univariate linear model
to each of the four data sets
, plot the results in four equally scaled windows, evaluate the results by computing
and check whether the caption provided by profil is justified by the data. For the calculation you may use the MATLAB program mat18_1.m.
Hint. A solution is suggested in the M-file mat18_exsol6.m.
- 7.
-
Continuation of Exercise 5, stock of buildings in Austria. Fit the model
and
. Further, analyse the increase of explanatory power through adding the respective missing variable in the models of Exercise 5, i.e., compute
and
as well as the average explanatory power of the individual coefficients. Compare with the result for data set number 5 in the applet Linear regression.
- 8.
-
The M-file mat18_ex8.m contains the mileage per gallon y of 30 cars depending on the engine displacement
, the horsepower
, the overall length
and the weight
of the vehicle (from: Motor Trend 1975, according to [19]). Fit the linear model
Hint. A suggested solution is given in the M-file mat18_exsol8.m.
- 9.
-
Check the results of Exercises 2 and 6 using the applet Linear regression (data sets 1 and 4); likewise for the Examples 18.1 and 18.8 with the data sets 8 and 3. In particular, investigate in data set 8 whether height, weight and the risk of breaking a leg are in any linear relation.
- 10.
-
Continuation of Exercise 14 from Sect. 8.4. A more accurate linear approximation to the relation between shear strength
and normal stress
is delivered by Coulomb’s model
where
and c [kPa] is interpreted as cohesion. Recompute the regression model of Exercise 14 in Sect. 8.4 with nonzero intercept. Check that the resulting cohesion is indeed small as compared to the applied stresses, and compare the resulting friction angles.
- 11.
-
(Change point analysis) The consumer prize data from Example 8.21 suggest that there might be a change in the slope of the regression line around the year 2013, see also Fig. 8.9. Given data
with ordered data points
, phenomena of this type can be modelled by a piecewise linear regression
and
are different,
is called a change point. A change point can be detected by fitting models
until a two-line model with the smallest total residual sum of squares
is found. The change point
is the point of intersection of the two predicted lines. (If the overall one-line model has the smallest
, there is no change point.) Find out whether there is a change point in the data of Example 8.21. If so, locate it and use the two-line model to predict the consumer price index for 2017.
- 12.
-
Atmospheric
concentration has been recorded at Mauna Loa, Hawai, since 1958. The yearly averages (1959–2008) in ppm can be found in the MATLAB program mat18_ex12.m; the data are from [14].
- (a)
-
Fit an exponential model
to the data and compare the prediction with the actual data (2017: 406.53 ppm).
Hint. Taking logarithms leads to the linear model
with
,
,
. Estimate the coefficients
,
and compute
,
as well as the prediction for y.
- (b)
-
Fit a square exponential model
to the data and check whether this yields a better fit and prediction.